While crystal balls belong to the realm of fantasy, predictive sales analytics offers the next best thing. This powerful tool allows businesses to enhance forecast accuracy, streamline decision-making, and ultimately drive growth and profitability. In this comprehensive guide, we’ll delve into what predictive sales analytics is, how it works, and the myriad benefits it can offer your business.
What You'll Gain from This Blog:
- Deep understanding of predictive sales analytics.
- Insights into its benefits and applications.
- Strategies to integrate predictive analytics into your sales workflows.
- Real-world examples and case studies demonstrating its impact.
What is Predictive Sales Analytics?
Predictive sales analytics is the practice of using data-driven algorithms and machine learning techniques to predict future sales outcomes. By analyzing historical data, user behaviors, market trends, and other variables, businesses can make educated forecasts about future sales performance. This predictive prowess equips companies with the ability to foresee potential challenges, uncover hidden opportunities, and make informed, preemptive decisions that can significantly affect their bottom lines. Through predictive sales analytics, businesses move from reactive decision-making to proactive strategies that drive sustained growth.
In addition to predictive capabilities, integrating sales reporting with predictive sales analytics provides a comprehensive view of both current and future performance. This combination allows businesses to not only understand past trends but also to anticipate future shifts, enabling more informed and strategic decision-making. Predictive sales analytics is not just about hitting sales targets. For example, it assists sales teams in identifying potential high-value leads and understanding customer lifetime value. This technology extends its reach beyond traditional sales forecasting, touching areas like customer relationship management, marketing optimization, and inventory control, hence streamlining various facets of business operations.
Boost revenue and maximize sales potential with gardenpatch's expert guidance. Their team is dedicated to aligning sales strategies for exceptional results. Click here to elevate your sales game!
How Predictive Sales Analytics Works
Predictive sales analytics involves a series of steps that transform raw data into actionable insights. Let’s break down these steps:
Data Collection and Integration
The first step in predictive sales analytics is gathering data from various sources. This can include CRM systems, social media platforms, website analytics, and third-party data providers. By integrating this data into a centralized repository, businesses can ensure they have a holistic view of their sales landscape. Data from these diverse sources provides a broader perspective, allowing more accurate, well-rounded predictions. For example, integrating social media analytics can reveal customer sentiment that traditional CRM data might miss.
Efficient data collection and integration also involve dealing with structured and unstructured data. Structured data from CRM systems and databases often need to be combined with unstructured data from emails, social media posts, and online reviews. This comprehensive integration process enriches the data pool, leading to deeper insights and more reliable predictive models.
Data Cleaning and Preparation
Raw data often contains inaccuracies, duplicates, or incomplete entries that can skew predictive models. Cleaning and preparing the data ensures that only high-quality information is used in the analysis, leading to more reliable outcomes. For instance, de-duplicating records and filling in missing values are essential steps in this process. Consistently clean data is fundamental to building models that businesses can trust.
Additionally, data cleaning involves transforming data into a suitable format for analysis. This might include converting textual data into numerical values through techniques like sentiment scoring or categorization. The cleaner and more prepared the data, the more accurate and insightful the predictive analytics outcomes will be.
Model Building
Once the data is clean, data scientists and analysts use machine learning algorithms to build predictive models. These models identify patterns and correlations within the data, creating a basis for accurate sales forecasts. Various algorithms, such as regression analysis, decision trees, and neural networks, can be employed depending on the complexity of the data and the specificity of the forecasting requirements.
Predictive models are continually evolving; as new data flows into the system, the models can be retrained and refined. This ongoing refinement ensures that the predictive models remain relevant and accurate over time, adapting to changes in market conditions and customer behaviors.
Validation and Testing
No model is perfect right from the start. Validation and testing phases are crucial to ensure that the model's predictions are reliable. Analysts use historical data to test the model's predictions against known outcomes, refining the algorithms as needed. This stage is essential to identify any discrepancies and improve the model's accuracy before it is deployed in a live environment.
Validation and testing also involve cross-validation techniques where the dataset is divided into multiple parts, testing the model's performance across different segments to ensure it generalizes well to unseen data. This rigorous testing phase is vital for instilling confidence in the model's predictive capabilities.
Deployment and Monitoring
After validation, the predictive model is deployed into production. It should be continuously monitored and refined based on new data and changing market conditions. Continuous monitoring helps identify any deviations in model performance and ensures that it adapts accurately to new trends and information. This dynamic adjustment is crucial for maintaining the effectiveness of predictive sales analytics in a continuously evolving marketplace.
Monitoring also involves performance metrics such as precision, recall, and the area under the curve (AUC), which help in understanding the model's strengths and areas for improvement. This systematic monitoring and updating ensure that the predictive model remains a valuable asset for the business over time.
The Benefits of Predictive Sales Analytics
Incorporating predictive sales analytics into your business strategy offers numerous benefits:
Improved Forecast Accuracy
Traditional sales forecasting methods can be prone to bias and subjectivity. Predictive sales analytics, on the other hand, uses objective data to generate highly accurate forecasts. This can significantly reduce the risk of overestimating or underestimating future sales. For example, rather than relying on salesperson intuition, predictive models analyze a diversity of data points to deliver precise forecasts. This leads to better planning, goal setting, and resource allocation.
Enhanced forecast accuracy helps businesses manage expectations and strategies more effectively. When forecasts are reliable, companies can align their operations, from production to marketing, more closely with anticipated demand, thereby minimizing waste and optimizing output.
Enhanced Decision-Making
Accurate forecasts empower sales managers and executives to make informed decisions. Whether it’s resource allocation, inventory management, or marketing strategies, predictive analytics provides the data needed to back up these critical choices. For instance, if the predictive model forecasts a dip in sales, the business can proactively adjust its marketing spend, offer promotions, or reallocate resources to areas with higher potential.
Enhanced decision-making extends beyond immediate tactical adjustments. Predictive analytics can inform long-term strategic decisions, such as entering new markets, launching new products, or restructuring sales teams. This data-driven approach ensures that every decision is aligned with the business's overall growth objectives.
Increased Efficiency and Productivity
Sales teams can spend less time on administrative tasks like data entry and report generation. Automated predictive models do the heavy lifting, allowing sales professionals to focus on what they do best—selling. By reducing time spent on mundane tasks, predictive analytics streamlines workflows and boosts productivity within the sales team.
Additionally, by prioritizing high-potential leads identified through predictive analytics, sales professionals can focus their efforts on opportunities that are more likely to convert, thereby increasing their overall efficiency and success rates. Predictive analytics helps sales teams work smarter, not harder.
Risk Mitigation
Predictive models can identify potential risks and opportunities before they become critical. By anticipating market fluctuations, customer churn, or supply chain disruptions, businesses can take proactive measures to mitigate these risks. For instance, if a model predicts a high likelihood of customer churn, the business can implement retention strategies to address the issue before it impacts the bottom line.
Risk mitigation through predictive analytics also involves scenario analysis, where different what-if scenarios are simulated to understand potential impacts and devise contingency plans. This proactive approach ensures that the business is better prepared to face uncertainties and make informed decisions under varying conditions.
Personalized Customer Experiences
By understanding customer behaviors and preferences, businesses can tailor their sales and marketing efforts to individual clients. This leads to higher customer satisfaction and loyalty. For example, predictive analytics can help identify which products a customer is likely to be interested in, allowing for personalized recommendations that enhance the customer experience.
Personalized customer experiences extend beyond product recommendations. Predictive analytics can inform personalized marketing campaigns, customized pricing strategies, and individualized customer service approaches. By delivering what the customer wants in a personalized manner, businesses can cultivate deeper relationships and foster enduring loyalty.
Integrating Predictive Sales Analytics into Your Workflow
So, how can businesses integrate predictive sales analytics seamlessly into their operations? Here are some actionable steps:
Start with Clear Objectives
Identify what you want to achieve with predictive analytics. Whether it's improving sales forecast accuracy, identifying high-potential leads, or optimizing your marketing strategy, having clear objectives will guide your efforts. Clear objectives help to focus resources and measure success more effectively. For instance, a company might set a goal to improve forecast accuracy by 15% within the next year using predictive analytics.
Setting clear objectives also helps in defining the scope and scale of the predictive analytics initiative. This ensures that the project remains aligned with broader business goals and delivers tangible results that contribute to the organization's overall success.
Invest in the Right Tools and Technology
There are numerous software solutions on the market that offer predictive sales analytics capabilities. Look for platforms that integrate easily with your existing CRM and data systems. Popular options include Salesforce Einstein Analytics, HubSpot CRM, and Microsoft Dynamics 365. Investing in the right tools ensures seamless data flow, efficient processing, and actionable insights.
In addition to investing in software solutions, consider the scalability and flexibility of the technology. Ensure that the chosen platform can grow with your business and accommodate increasing data volumes and complexities. Integration capabilities are crucial for creating a unified data ecosystem that supports comprehensive predictive analytics.
Hire or Train Skilled Personnel
Predictive analytics requires specialized skills in data science, machine learning, and statistical analysis. You can either hire new talent or invest in training for your existing team to build these competencies. Having skilled personnel ensures that the predictive models are built, validated, and maintained effectively.
Investing in ongoing education and professional development is equally important. The field of data science and machine learning is continuously evolving, and staying updated with the latest techniques and tools can provide a competitive edge. Whether through hiring experts or developing in-house talent, skilled personnel are essential for the success of predictive analytics initiatives.
Foster a Data-Driven Culture
For predictive analytics to be effective, it needs to be embraced at all levels of the organization. Encourage a culture where decisions are driven by data and insights rather than gut feelings and intuition. This cultural shift requires leadership buy-in and consistent communication about the value of data-driven decision-making.
Creating a data-driven culture also involves democratizing access to data and insights. When employees at all levels have access to relevant data and understand its implications, they are more likely to embrace data-driven decision-making. Training sessions, workshops, and regular updates can help foster this culture effectively.
Continuously Monitor and Refine
Predictive models are not set-it-and-forget-it solutions. They need continuous monitoring and refinement based on new data and changing business conditions. Establish a process for regularly reviewing and updating your models to ensure they remain accurate and relevant. This dynamic approach ensures that the predictive models adapt to evolving market trends and continue to deliver valuable insights.
Continuous monitoring and refinement also involve leveraging feedback from sales teams and other stakeholders. By incorporating practical insights and real-world feedback, predictive models can be fine-tuned to better meet the business's needs and drive more effective outcomes.
Challenges and Considerations
While the benefits of predictive sales analytics are substantial, there are also challenges that businesses must navigate:
Data Quality and Integration
Poor data quality can lead to inaccurate predictions. Ensuring that all data sources are accurate, comprehensive, and integrated is crucial for reliable analytics. Investing in robust data management practices and technologies is essential to maintain high data quality and achieve meaningful insights.
Data integration also presents challenges, especially when dealing with disparate data sources and formats. Establishing a unified data architecture and leveraging data integration tools can streamline this process and enhance the overall effectiveness of predictive analytics.
Complexity and Cost
Implementing predictive sales analytics can be complex and costly. It requires investment in technology, skilled personnel, and continuous model maintenance. Businesses must weigh the costs against the potential benefits and ensure that they have the necessary resources and commitment to support the initiative.
Managing the complexity involves establishing clear workflows, defining roles and responsibilities, and adopting project management best practices. Effective management of complexity and costs ensures that the predictive analytics initiative is sustainable and delivers long-term value.
Change Management
Adopting a data-driven approach may require a cultural shift within the organization. Change management strategies are essential to get buy-in from all stakeholders and ensure successful implementation. This involves clear communication, training programs, and aligning the initiative with broader business goals to gain support and commitment.
Successful change management also depends on demonstrating quick wins and tangible benefits early in the process. When stakeholders see the positive impact of predictive analytics, they are more likely to support and champion the initiative, leading to broader acceptance and integration across the organization.
Conclusion
Predictive sales analytics is revolutionizing the way businesses approach sales forecasting and decision-making. By leveraging data-driven insights, companies can improve forecast accuracy, enhance operational efficiency, and deliver personalized customer experiences. The journey to integrating predictive analytics may come with challenges, but the rewards far outweigh the obstacles. Start with clear objectives, invest in the right tools and talent, and foster a culture that embraces data-driven decision-making. With predictive sales analytics, your business can turn uncertainty into opportunity and drive sustainable growth.
Ready to harness the power of predictive sales analytics for your business? Start by assessing your current data landscape and identifying your key objectives. Consider consulting with experts in the field or investing in training for your team. The future is data-driven, and the time to act is now.
Remember, the essence of effective content creation lies in delivering valuable, actionable information to your audience, all while maintaining a compelling narrative built on verified data and real-world examples. By following these principles, you can create a blog that not only captures attention but also drives meaningful engagement and business results.
Popular Insights:
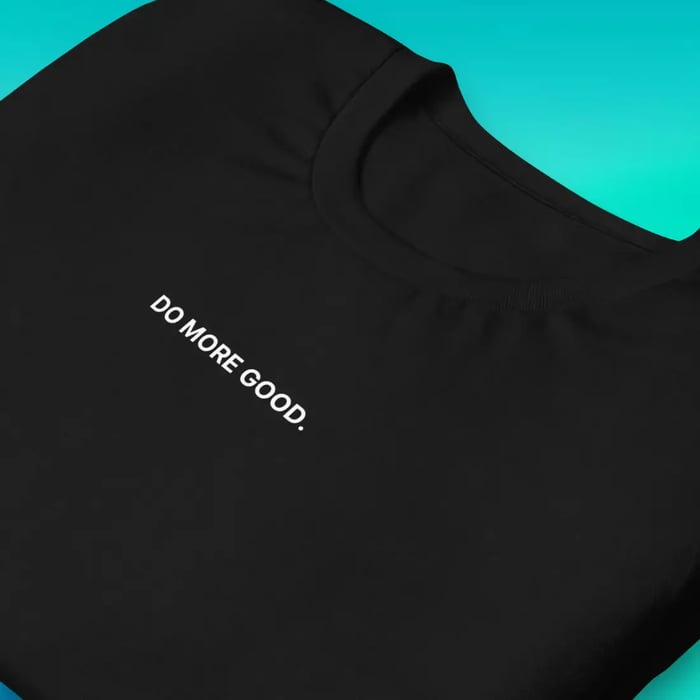
Do More Good. T-Shirt | V1
$30.00Shop with Purpose at Impact Mart! Your Purchase Empowers Positive Change. Thanks for Being the Difference!